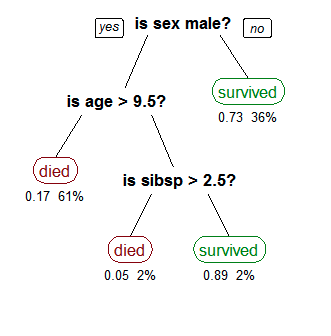
Random forest
Until a few years ago the Random Forest was considered as one of the most powerful machine learning algorithms. As you can guess from its name, a random forest model contains many decision trees. In order to train these trees, the algorithm samples the data randomly with replacement creating several subsamples and trains one tree on each of the subsamples. Then it combines the prediction of the trees, for example by using majority vote for a classification problem, and averaging the predictions for a regression problem.
Boosting
Boosting algorithms are newer and even more powerful tools than Random Forest. While Random Forest builds trees in parallel, boosting algorithms build the trees one after the other, taking into account the weakest points of the previous models, and creating a model that strengthens those points in the next step, converting weak learners into a strong one as a result. The two most popular methods both use gradient boosting. LightGBM was developed by Microsoft (https://github.com/microsoft/LightGBM), while XGBoost (https://github.com/dmlc/xgboost) was developed as an open source project. Both algorithms are fast, implement parallel processing, and can be used for a great variety of problems.
Interpreting ensemble methods
As mentioned above, a huge advantage of tree-based methods is their interpretability, that it’s possible to understand why the algorithm predicts what it predicts. All the algorithms we discussed in this post provide tools to examine the importance of each of the input features. There are also several external tools that help to interpret your model, such as xgboostExplainer for the R language (https://github.com/gameofdimension/xgboost_explainer) and LIME (https://github.com/marcotcr/lime) or SHAP (https://github.com/slundberg/shap) for Python. These tools can help you discover deeper connections between the features, and refine your models; you can even use them to create illustrations that help explain how your model works to non-experts. The image below shows a figure created with SHAP using a model for predicting the survival of passengers of the Titanic (https://meichenlu.com/2018-11-10-SHAP-explainable-machine-learning/). It shows that females, first and second class passengers, and children had the best chance of survival.